Proprietary data formats are killing your AI initiative
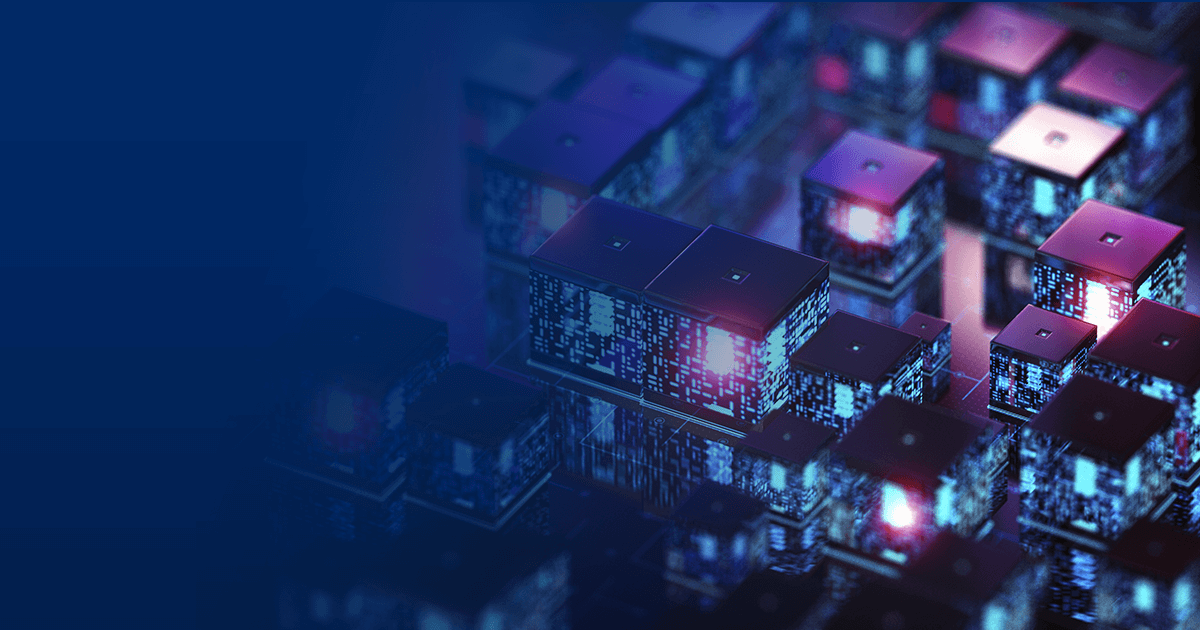
Legacy data architectures and a do-it-yourself approach to data management present key obstacles to achieving your AI goals and improving scientific outcomes. But unfortunately, they’re not the only impediments. A lack of harmonization among data formats and metadata can grind your AI journey to a complete halt.
Your organization might have hundreds or thousands of scientific instruments and applications. And they produce data, often unstructured, in vendor-specific proprietary formats, with unique metadata terms and organizational schemes.
These formats have limited accessibility outside of each vendor’s walled garden. For example, you might be unable to access data produced by a chromatographic instrument from within an application from another vendor without first doing manual data transformation. Want to analyze or visualize experimental results from multiple instruments? You’ll need to harmonize data so that it’s all accessible from within the analytics or visualization software of your choice.
Preparing the large-scale datasets for AI applications is a much bigger task. But until you can harmonize all that data, you won’t be able to take advantage of the full potential of AI.
Adopting a single, open, and vendor-agnostic format for scientific data could go a long way toward reducing this barrier in the AI journey. So, why hasn’t the life science industry successfully established a standard? And how does metadata complicate the work of harmonizing data from various instruments and applications?
Attempts at standards
There have actually been a number of efforts to create data standards in the life science industry. But none of these standards has been widely adopted as of today. For example:
- The Allotrope Foundation developed the Allotrope Data Format (ADF)—a “federation of standards” designed to store large, complex datasets in a single file. For many organizations, it was too complicated. Subsequently, it created the Allotrope Simple Model (ASM) to standardize the structure of instrument data. (Learn how to create data in the ASM at scale with TetraScience.)
- Analytical Information Markup Language (AnIML) is a standard for storing and sharing analytical chemistry and biological data. While it originated from initiatives more than two decades ago, it never gained much traction.
- The Standardization in Lab Automation (SiLA) consortium is working to standardize software interfaces specifically for working with robotic automation. But the consortium isn’t providing a true solution for harmonizing the actual data for other data usages.
- The Pistoia Alliance created the Unified Data Model (UDM) project to produce an open data format for storing and exchanging experimental information about compound synthesis and testing.
Moreover, individual vendors have attempted to establish their own “standards,” which are really just proprietary formats disguised as standards. They are clearly not open or vendor-agnostic formats.
In parallel, an international consortium of scientists and organizations introduced FAIR data principles to optimize reuse of data. FAIR data is findable, accessible, interoperable, and reusable. These principles provide a strong guide for enhancing data reuse, but they are still only principles. They do not establish a single industry-standard format.
Standards inertia
There are several reasons why standardization efforts have faltered or failed. And those same reasons have restrained interest in launching new standards projects. Overall, there are few incentives for life science technology companies to establish new standards, adopt standards created by other organizations, or endorse open, vendor-agnostic data formats
- The quest for competitive advantage: Vendors develop proprietary data formats that support their particular capabilities—capabilities that provide them with competitive advantages. Vendors typically do not want to adopt or help establish open, industry-wide standards that might in any way diminish the benefits of their unique, differentiating capabilities.
- Legacy designs: Much of the instrument control and data acquisition software available today was initially built on legacy technology and meant to be installed on lab PCs. Each application was designed to give scientists all the functionality to complete their work without leaving that application. Vendors have no motivation to develop an open, vendor-agnostic data format or push for standards.
- Customer retention: For instrument and application companies, vendor lock-in is generally a good thing. Maintaining proprietary data formats helps them retain their existing customers. Vendors want to keep customers within their walled gardens, binding customers to their particular ecosystem.
- Complexity: Of course, it’s not just that vendors want to preserve their investments and retain their customers. Developing industry-wide standards is also hard work. Different instruments and modalities produce different bits of information—it is difficult to find one size (that is, one data format) to fit all. The efforts of consortia to create standards have shown how challenging it is to achieve compromise among multiple parties.
If the industry could establish a standard data format, biopharma organizations would still need to get their data to conform to that standard, which would not be an easy task. But without a standard, biopharma companies are stuck with multiple data silos. Data remains trapped in vendor-specific data formats within on-premises environments. To use that data with AI applications, an organization would need to invest significant time and money for harmonization—or they will need to find a commercial solution purpose built to solve standardization challenges.
Metadata harmonization
Harmonizing data formats is essential for enabling data liquidity and producing the large-scale datasets needed for AI. But harmonization can’t stop with the data format—it must also be applied to the taxonomies and ontologies in the metadata.
A data taxonomy defines elements and their organization. A data ontology describes the relationships among elements. Together, the taxonomy and ontology form a vocabulary that captures key scientific information about samples, materials, equipment, results, and more. This vocabulary is vital for finding, interpreting, analyzing, and assembling data—and using the right data for AI algorithms.
Instruments, applications, and users often use their own terminology and add unique contextual labels and other metadata. As your lab collects data from more and more sources, you are likely to be left with multiple terms to describe the same data. You might even see this inconsistency if you were to compare metadata from different departments, sites, or regions within your organization—or even between scientists.
Unless you harmonize taxonomies and ontologies, it will be difficult for users to find, compare, and reuse data from all of your different sources—and it will be impossible to assemble that data into the large-scale datasets needed for advanced analytics and AI.
This metadata harmonization work must be an ongoing effort because taxonomies and ontologies can evolve. For example, the Allotrope Taxonomies and Ontologies included with the Allotrope Data Format were initially based on existing vocabularies but then grew to several thousand terms and properties as more companies began using the data format. Metadata flexibility is helpful as organizations refine their workflows and use cases over time—but these evolving taxonomies and ontologies must be maintained.
Understanding the Scientific AI gap
There’s no question that biopharma executives have ambitious goals for using AI to accelerate delivery of breakthrough therapeutics, streamline manufacturing, improve quality control, and more. They are investing heavily in AI initiatives and expect results fast.
Unfortunately, most companies are still far away from meeting AI objectives. Along with legacy architectures and a DIY approach to data integration, a diversity of data formats and metadata vocabularies present formidable obstacles on the AI journey. Organizations need to dramatically simplify data and metadata harmonization efforts before they can start realizing the tremendous potential of AI in science.
Learn more about the AI gap in the white paper, "The Scientific AI Gap."